Methodological advice on Family Occupation and Education Index (FOEI)
This report was originally published 05 November 2013.
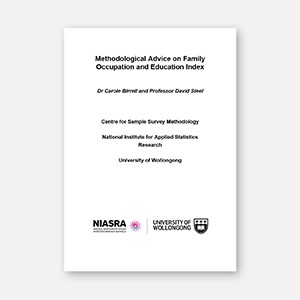
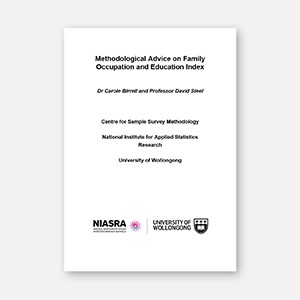
Summary
The Family Occupation and Education Index (FOEI) is a school-level socio-economic index developed by the NSW Department of Education and Communities (DEC). The FOEI is based on school-level regression analysis of the relationship between the average of standardised students’ achievement scores obtained from NAPLAN results and parental background variables, which are the level of highest school education, highest non-school education and occupation. In developing the 2013 FOEI, the National Institute for Applied Statistics Research Australia at the University of Wollongong has been contracted to review and provide external validation of the FOEI methodology due to the proposed use of the FOEI data in resource allocation.
For the purpose of this review, a sample of 2012 data was provided by NSW DEC to NIASRA. The sample data included approximately 50% of schools with all students at the selected schools. Data included, for each de-identified student record, student year of schooling, gender, Aboriginal status, reported and standardised NAPLAN reading and numeracy results, parent education and occupation variables, and a set of school and community variables derived from the 2011 ABS census.
In developing the 2013 FOEI several technical issues associated with missing data and regression analysis have been considered and the following approaches are recommended to cope with them.
Imputation
A. Use a model-based multiple imputation approach to reduce bias in FOEI arising from missing parental background data
Data on parents can be missing. This missing data affects the estimation of the regression function and the calculation of FOEI scores for individual schools. A model-based multiple imputation approach – multiple imputations by chained equations (MICE) – is recommended to deal with missing data. This approach uses the relationships between the variables in the observed data to impute plausible values for the missing data. This is done multiple times (M=10) to enable valid estimates of uncertainty accounting for both the regression model estimation and the imputation itself. This is a widely adopted and flexible approach that allows the full use of the observed data for many variables and can be implemented using readily available statistical software (White et al, 2011). As with any imputation approach the method is based on some assumptions, including the assumption that conditional on the observed data the unobserved data are missing at random. The missing at random (MAR) assumption is less restrictive than the assumption of Missing Completely at Random (MCAR) which is assumed if complete cases, that is students for whom all parental data are available, only are used in the analysis. It is possible that the mechanism of missingness is Missing Not at Random (MNAR), however, the use of a large number of explanatory variables in the imputation model should assist in moving closer to the MAR assumption and therefore reduce any possible bias (White et al, 2011).
B. Use as many relevant variables as possible to help the imputation process
It is recommended that the parental variables are used along with the standardised student achievement scores (when available), ATSI status, school remoteness and a set of community variables derived from the 2011 ABS census including levels of education and occupation of persons in the same statistical area (SA1) as the student’s address. These community census variables consist of percentages of people/families and were calculated for all people or all families in the same area as the student’s address.
The imputation does not explicitly use the school indicator variable, although the imputation uses the community variables and therefore reflects some characteristics of the local community. Imputation taking into account the nesting structure of the data (i.e., students are clustered in schools) was not adopted because of concerns about the stability of relationships based on small numbers of responding cases in many schools. Some of the larger schools would have had sufficient cases to consider imputation within the school, but then the models of imputation would have differed across schools, which was considered unreliable.
C. Use different imputation models for one parent and two parent students
Some students have one parent and some have two parents in the data file. To allow for the use of information on the second parent, the imputation is conducted separately for cases where there are data for two parents and cases with data for only one parent. The imputation for one parent and two parent cases was done separately, on the basis of the department’s research that shows the relationship between the parent background variables is different for the one parent variables and the equivalent variables in the two-parent data file. There is a sufficient number of students from both one and two parent families to enable the relationships amongst parent variables to be estimated separately for the two groups of students.
D. Use multiple years of NAPLAN data to maximise the number of students with available standardised achievement data to help the imputation process
For example, in the sample data provided, standardised NAPLAN reading and numeracy results for students in Years 3, 5, 7 and 9 in 2012 were used in addition to matched 2011 results for students in Years 4, 6, 8 and 10 in 2012. This allowed standardised achievement data to be used, in the imputation process for the review, for Years 3 - 10 for students in 2012.
E. Use a different imputation model for students without NAPLAN achievement scores
Students who were exempted from NAPLAN or from cohorts for which NAPLAN data does not exist in the review data set (e.g. students in Kindergarten, Year 1, and Year 2) are included in the imputation with other students but student achievement scores are unavailable and therefore not used in the imputation.
Regression Analysis
F. The FOEI regression model proposed by DEC can be implemented using the following approaches:
- The dependent variable in the regression analysis is based on the most recent observed student achievement scores. For the 2013 FOEI, these are the 2012 NAPLAN reading and numeracy scores, standardised and averaged, for students now in Years 4, 6, 8 and 10 in 2013.
- The explanatory variables are the school level parental background variables (i.e. the percentages of parents in each category of school education, non-school education and occupation). These are calculated using parental background data for all students in the school in 2013, including the imputed values for missing data.
- The regression analysis uses a dependent variable based on observed 2012 student achievement data for students in Year 4, 6, 8 and 10 in 2013, while the explanatory variables are based on parental background variables for all students attending the school in 2013. The regression equation is designed to reflect the relationship between achievement in the most recent year for which achievement data are available and the parental background of students. Since calculation of the FOEI score for a school is based on the parental background of all students, estimation of the regression function also uses these as the explanatory variables.
- The review considered the inclusion of ABS community variables in the regression analysis. Previous regression analysis performed by the DEC suggested no appreciable additional predictive power is added if the community level variables are used in the school-level regression analysis. However, community level variables are useful information to help impute for missing parental data.
G. Weighting parental information for students in one parent families
In the regression estimation and in the production of the FOEI score the school-level parental variables effectively average the characteristics of the parents in a two-parent case. In order for each student’s family to count equally towards the school FOEI score, the calculation of the school- level variables assigns a weight of 2 to single parents and a weight of 1 to each parent in a two-parent family.
H. Robust regression is the regression technique recommended to construct FOEI scores to deal with outliers
Analysis of residual patterns from ordinary least squares (OLS) and comparisons of OLS, weighted least squares (WLS) and robust regression models show that robust regression is a technique that is effective in reducing the influence of outliers on the regression estimates. The majority of outliers from the OLS analysis are selective schools and small schools. However not all small schools are outliers; in fact the majority of small schools fit the regression model reasonably well. Robust regression reduces the weight for the outliers, i.e., schools that have large residuals. Using robust regression appears to adequately account for both selective schools and the small schools with large residuals, and is recommended.